Features¶
ML-Ensemble a Python library for memory efficient parallelized ensemble learning. In particular, ML-Ensemble is a Scikit-learn compatible library for building deep ensemble networks in just a few lines of code.
ensemble = SuperLearner().add(estimators)
ensemble.fit(xtrain, ytrain).predict(xtest)
A computuational graph approach to ensembles¶
Ensembles are built on top of a computational graph, giving maximal design freedom. Graphs are straightforward to optimize for speed, and automatically minimize memory consumption. Ready-made ensemble classes allow you to build optimized ensembles in just a few lines of code. The low-level API gives you full control of the ensemble network and the computational procedure to build virtually any type of ensemble, including dynamic and recursive features.
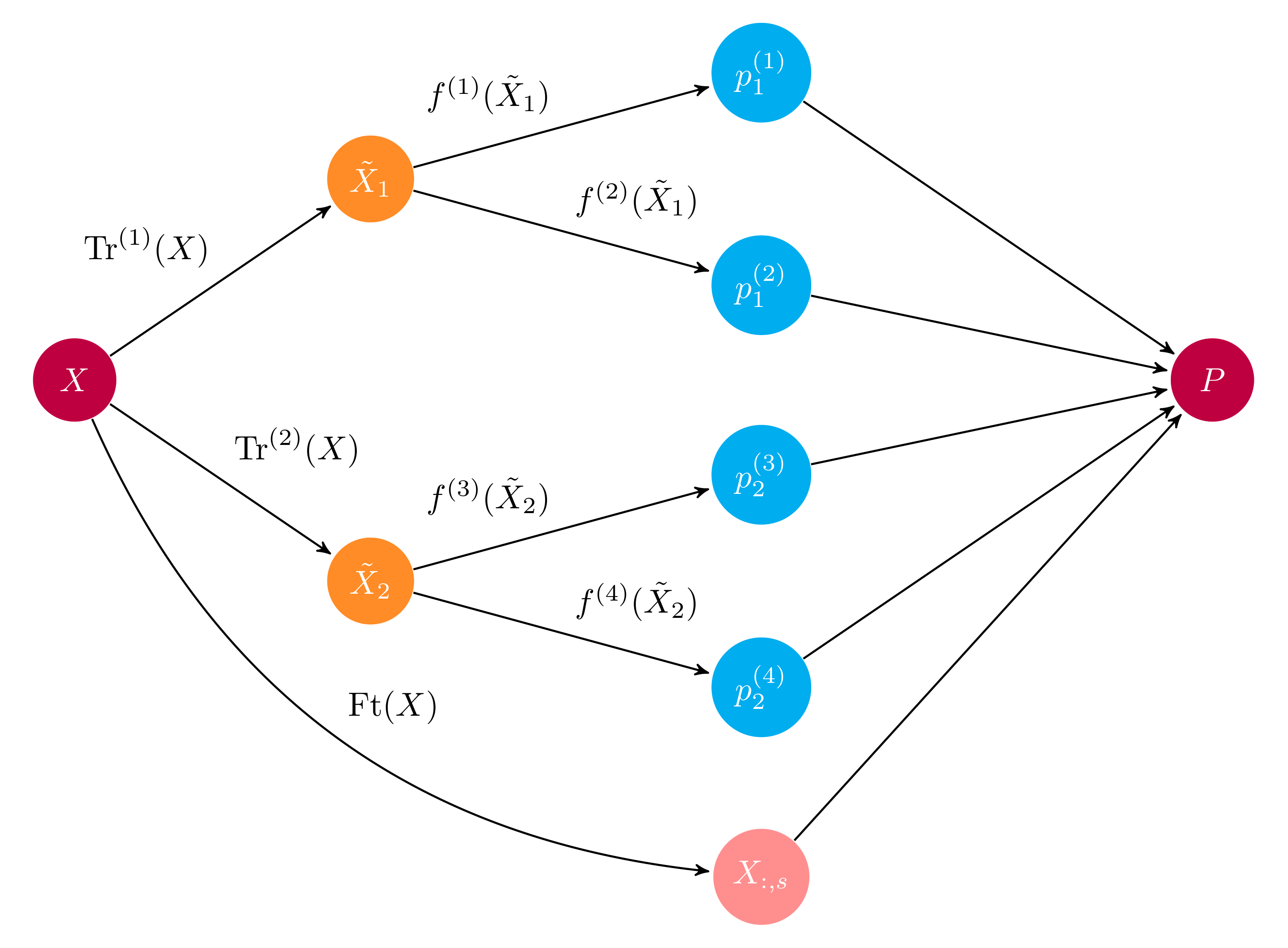
A typical computational graph. An input \(X\) is differentialy preprocessed via tranformers \(\textrm{Tr}^{(j)}\) before base learners \(f^{(i)}\) generate predictions \(p^{(i)}_j\). Predictions are concatenated along with any propagated features from the input (\(X_{:, s}\)) to form the output matrix \(P\).
Ease of Use¶
Simply call the add
method with a set of estimators to stack a layer on an ensemble.
Ensembles are Scikit-learn compatible estimators. No matter how complex the
ensemble, to train with the fit
method:
ensemble = Subsemble()
# First layer
ensemble.add(list_of_estimators)
# Second layer
ensemble.add(list_of_estimators)
# Final meta estimator
ensemble.add_meta(estimator)
# Train ensemble
ensemble.fit(X, y)
Memory Efficient Parallelized Learning¶
ML-Ensemble is designed to maximize parallelization at minimum memory footprint and is designed to be thread-safe. It can fall back on multiprocessing seamlessly and use shared memory to avoid array copying and serialization. For more details, see the memory benchmark.
Differentiated preprocessing pipelines¶
ML-Ensemble offers the possibility to specify a set of preprocessing pipelines that map to a specific group of estimators in a layer. Implementing differentiated preprocessing is straightforward and simply requires a dictionary mapping between preprocessing cases and estimators:
ensemble = SuperLearner()
preprocessing = {'pipeline-1': list_of_transformers_1,
'pipeline-2': list_of_transformers_2}
estimators = {'pipeline-1': list_of_estimators_1,
'pipeline-2': list_of_estimators_2}
ensemble.add(estimators, preprocessing)
Dedicated Diagnostics¶
ML-Ensemble is equipped with a model selection suite that lets you compare several models across any number of preprocessing pipelines in one go. In fact, you can use an ensemble as a preprocessing input to tune higher levels of an ensemble. Output is directly summarized in table format for easy comparison of performance.
>>> evaluator.results
test_score_mean test_score_std train_score_mean train_score_std fit_time_mean fit_time_std params
class rf 0.955357 0.060950 0.972535 0.008303 0.024585 0.014300 {'max_depth': 5}
svc 0.961607 0.070818 0.972535 0.008303 0.000800 0.000233 {'C': 7.67070164682}
proba rf 0.980357 0.046873 0.992254 0.007007 0.022789 0.003296 {'max_depth': 3, 'max_features': 0.883535082341}
svc 0.974107 0.051901 0.969718 0.008060 0.000994 0.000367 {'C': 0.209602254061}